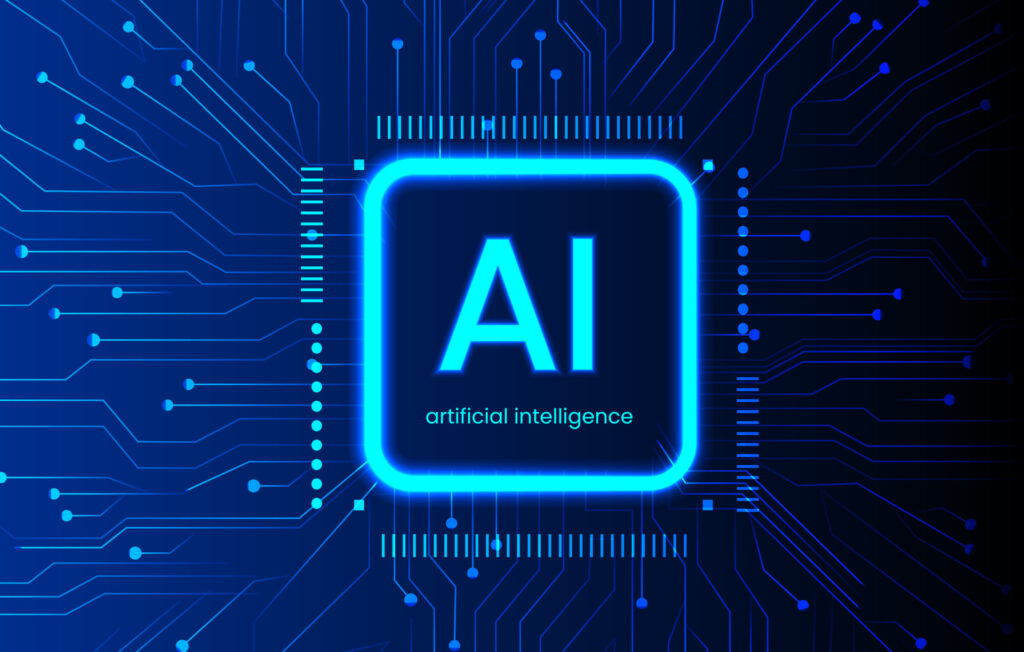
Integrating Generative AI in Quality Engineering: Challenges and Solutions in 2025
Introduction
Generative AI, a groundbreaking technological advancement, has ushered in a new era for the field of Quality Engineering, fundamentally reshaping the way software development teams approach the critical tasks of testing, validation, and overall quality assurance. This transformative technology has the potential to enhance the efficiency, accuracy, and cost-effectiveness of Quality Engineering practices, leading to greater innovation and higher-quality software products.
The remarkable capabilities of generative AI models, which can produce human-like text, images, and even code with unprecedented accuracy, have facilitated their integration into a diverse range of Quality Engineering applications. According to (Oniani et al., 2023), the use of generative AI in fields such as collaborative writing and software development has been rapidly expanding, as industries recognize the potential to streamline and optimize their processes.
Key Challenges in Generative AI for QE (2024)
1. Reliability of AI-Generated Outputs
However, despite their power, Generative AI systems are not error free. This gives them the ability to create test cases that ignore important edge cases, or result in imprecise answers. Because of this limitation, there exist significant risks in industries like healthcare where undetected flaws can lead to safety hazards, or finance where inaccurracies could cause regulatory non compliance.
2. Dependence on High-Quality Data
Trained on quality data, AI models can be powerful. Unchosen or poorly curated datasets can do more harm than good, skewing the results away from the reliability of AI driven quality assurance. For organizations trying to get as much as possible out of Generative AI, one persistent challenge is producing comprehensive and accurate datasets.
3. Infrastructure Limitations
Legacy IT systems are often lacking the computational power and flexibility necessary to host AI integrations. It can be expensive and time consuming to upgrade these infrastructures, with many concerns over return on investment and operational disruption in the process of transitioning.
4. Ethical and Regulatory Compliance
Despite the rapid growth of Generative AI, the speed at which Generative AI is developing in terms of mechanism and application has outpaced the development of regulatory frameworks, leaving organisations in murky uncertainty regarding whose responsibility accountability will be and how transparently this new generation of technology will have to be governed. Companies competing in heavily regulated sectors face a serious challenge with this gap.
5. Workforce Skills Gap
One of the biggest stumbling blocks to ultimately successful AI integration is a lack of people skilled to bridge the gap between AI and quality engineering. Slow adoption of advanced technologies — and failure to fully reap the benefits of investments in Generative AI — is due to this skills gap.
Emerging Issues for Generative AI in 2025
1. Scaling AI Across Complex Systems
The casings of AI in multi cloud and interconnected systems present complexities regarding maintaining uniformity in performance. Such architecches demand new strategies of scalability.
2. AI Drift
AI drift, the gradual degradation in model performance owing to changes in data patterns or environments, will be an issue. To solve this will require frequent retraining and consume extensive computational resources resulting in both increased cost and efficiency.
3. Cybersecurity Threats
Adversarial attack is inherent to AI systems. Because vulnerabilities exist in the Generative AI models, malicious actors will be able to exploit these vulnerabilities to break into sensitive data or cause the operations to crash.
4. Regulatory Maturity
By the time regulatory bodies have figured out how to govern AI, those requirements will tighten into compliance. To keep up with these requirements, organizations will have to spend on sophisticated monitoring instruments and clear processes.
5. Ethical Biases
Efforts to fix a bias for AI systems have been made, but there remains challenge with, for example, recruitment, loan approvals and healthcare. We will continue to demand innovation and vigilance around fair and inclusive affairs.
6. Human-AI Collaboration
It will be important to find a balance between human oversight and AI automation. If roles and responsibilities are not defined well, it won’t help with workflow disruption and inefficiencies that will affect the benefits of integrating AI.
Proven Strategies to Overcome Challenges
1. Ensuring Reliable AI Outputs
- Validation Frameworks: Establish rigorous frameworks to test and validate AI-generated outputs.
- Edge Case Focus: Collaborate with domain experts to identify and address critical edge cases that AI may overlook.
2. Improving Data Quality
- Governance Practices: Implement robust data governance protocols to ensure the integrity of training datasets.
- Synthetic Data: Leverage AI-generated synthetic data to fill gaps in existing datasets, improving model performance and reducing biases.
3. Optimizing Infrastructure
- Cloud Solutions: Transition to scalable, cloud-based platforms that can handle the computational demands of Generative AI.
- Green AI Models: Adopt energy-efficient AI architectures to minimize environmental impact while enhancing performance.
4. Ethical Compliance
- Proactive Adaptation: Stay informed about emerging regulations, such as the EU AI Act, and prepare for proactive compliance.
- Transparent Systems: Use explainable AI (XAI) tools to ensure transparency and build stakeholder trust.
5. Bridging the Skills Gap
- Upskilling Workforce: Invest in comprehensive training programs to equip teams with AI and QE expertise.
- Cross-Team Collaboration: Foster collaboration between engineers, data scientists, and business leaders to bridge knowledge gaps and align objectives.
6. Mitigating AI Drift
- Model Monitoring: Deploy continuous monitoring systems to track AI performance and detect early signs of drift.
- Frequent Updates: Schedule regular model retraining to adapt to evolving data environments and maintain accuracy.
7. Strengthening Cybersecurity
- AI-Specific Protocols: Introduce tailored encryption and adversarial attack detection mechanisms to protect AI systems.
- Threat Modeling: Regularly assess potential vulnerabilities and update security measures to counter emerging threats.
8. Addressing Bias
- Detection Tools: Utilize advanced tools to identify and mitigate biases in datasets and AI models.
- Diverse Teams: Incorporate a wide range of perspectives during AI model development to enhance fairness and inclusivity.
9. Enhancing Human-AI Collaboration
- Role Clarity: Define specific roles and responsibilities for human operators and AI systems to streamline workflows.
- User-Friendly Tools: Design intuitive AI interfaces that empower users to interact effectively with automated systems.
Conclusion
This is a paradigm shift in Quality Engineering and generative AI opens new avenues for improving process and consistent scaling while at the same time driving more innovation. Nevertheless, these benefits involve some challenges that call for strategic foresight and proactive steps. Generative AI has the potential to do enormous good for the world, automating time-consuming, repetitive tasks, fostering creativity, and creating efficiencies that improve lives both individually and collectively, but by addressing the risk of AI drift, ethical biases, and cybersecurity threats organizations can take advantage of the full power of Generative AI while preserving trust and compliance.
This rapid change demands a commitment to learning, collaboration and innovating continuously. Whether you’re starting to integrate AI or trying to improve the work you’re already doing, these strategies can be your roadmap to making it work.
References:
- Adopting and expanding ethical principles for generative artificial intelligence from military to healthcare 2023 https://www.nature.com/articles/s41746-023-00965-x
- The Impact of Artificial Intelligence on Quality and Safety 2020 https://journals.sagepub.com/doi/10.1177/2192568219878133
- Generative AI and jobs: a global analysis of potential effects on job quantity and quality 2023 https://researchrepository.ilo.org/esploro/outputs/encyclopediaEntry/995326516102676