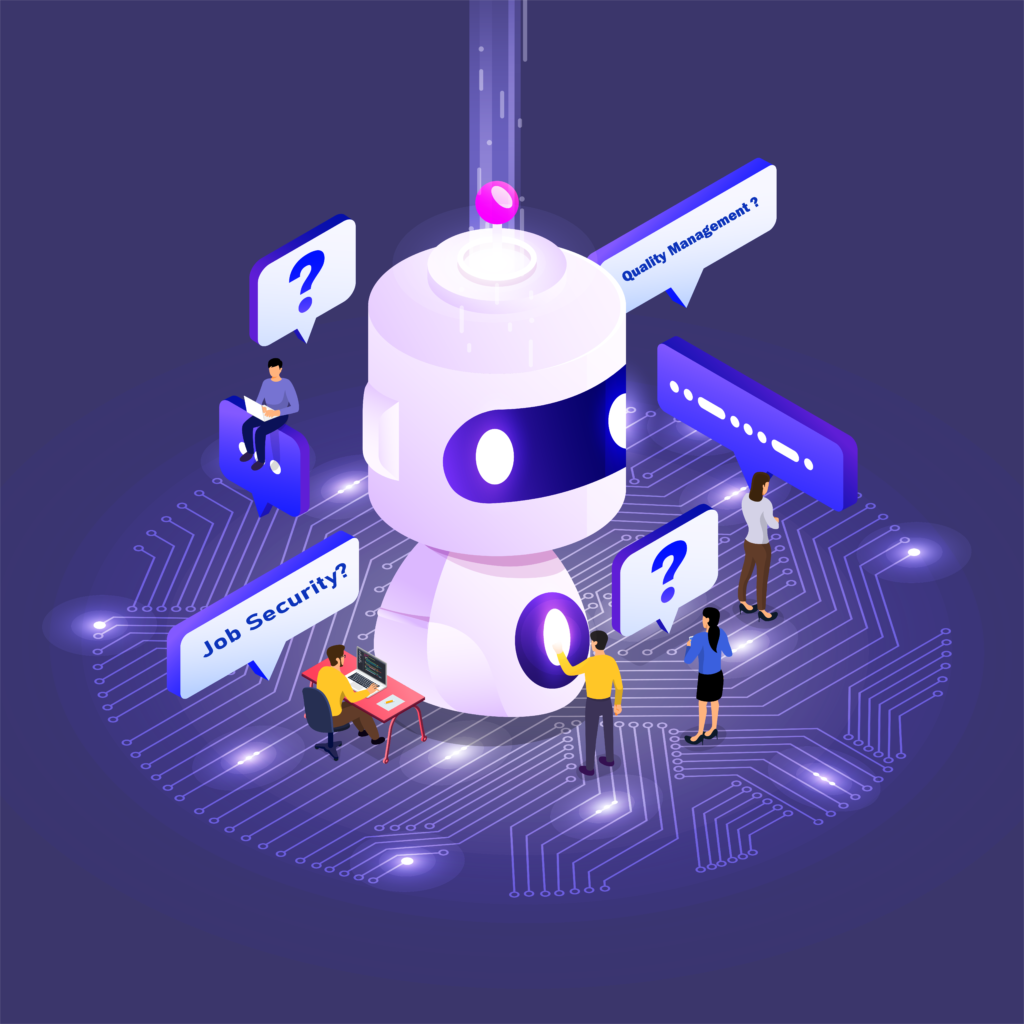
Debunking AI Myths in Quality Management: Essential Insights for 2025
Introduction: Understanding AI’s Role in Quality Management
Organizations are revolutionized by artificial intelligence when it to how to monitor, control, monitor, and improve their process. AI is changing the traditional quality assurance role, from predictive maintenance in manufacturing to compliance in service delivery. However, myths surrounding it can cloud it’s transformative potential and hinder effective adoption or fan resistance among professionals. To enable AI to really play its part in quality management, understanding these myths is essential.
According to the incorporation of AI into data management processes can result in significant improvements in decision-making by enhancing the reliability and accuracy of the underlying data (Khatib & Falasi, 2021).
As AI-powered technologies continue to revolutionize diverse industries, the field of quality management has been at the forefront of this transformative shift. Despite the immense potential of AI to enhance quality assurance processes, persistent myths and misconceptions have often impeded its wider integration within the industry. The advent of artificial intelligence (AI) is changing quality management: bringing its unparalleled efficiencies and capabilities to business operations at unprecedented levels. But this quick adoption comes with myths and misconceptions that can cause people to be afraid of the wrong things or simply have too many expectations for what is and what isn’t possible. In this article these myths are debunked, fact from fiction, and actionable insights for future proofing quality management careers in a fast moving landscape are offered.
Myth 1: AI Will Replace Quality Management Professionals
The Misconception: However, many claim AI will take over quality management so much that professionals are no longer needed.
The Reality: AI works better when it is teamed up with humans. It’s great at processing huge amounts of data, identifying patterns, and performs repetitive tasks. But quality management is something that involves making decisions, ethical considerations, and complex, complicated problem solving, which is better with human insight. Suppose that AI was to flag a pattern of defects in a production line, but the ‘cause’ in each case is a professional deciding whether the root cause is in training, materials, or equipment malfunction.
Myth 2: AI Guarantees Error-Free Quality Processes
The Misconception: Human error is eliminated by AI, resulting is flawless quality outcomes.
The Reality: Artificial intelligence can eliminate some types of errors, but if not monitored it adds to other types of errors. First, data is only as effective as the system it powers. For instance, biased or incomplete datasets can be the cause of bias or skew of AI driven analyses. This is only possible with quality professionals who are validating the AI outputs and if the decisions are in line with organization’s goals and values.
Myth 3: AI Implementation Is Quick and Effortless
The Misconception: Installing software to deploy AI in quality management is as simple as deploying software.
The Reality: There must be time, planning, and taking the integration and change management seriously if AI adoption is to succeed. It is organizations’ job to review how ready their data is, investing in the infrastructure, and training the people alongside the AI tools. In addition, AI capabilities are aligned with business objectives so they drive measurable improvement.
Myth 4: AI Solves All Challenges Without Human Oversight
The Misconception: Every quality management challenge can be handled by AI, independently.
The Reality: Contextual understanding is what AI lacks, and it isn’t capable of navigating complex or ambiguous scenarios. For example, AI might spot a trend of customer complaints, but human understanding of cultural nuances, market dynamics is needed to get that pattern. It is only through quality management professionals that we ensure that AI outputs are interpreted as well as acted upon.
Myth 5: Continuous Improvement Becomes Obsolete With AI
The Misconception: Without traditional quality improvement methodologies AI significantly reduces the need.
The Reality: Because Continuous improvement frameworks like Lean and Six Sigma are still important. AI enriches these methodologies by supplying real time dataset learning and predictive analytical but the testing–feedback–refinement cycle of iteration is integral. Human oversight maintains that improvements remain sustainable and on agenda.
Myth 6: AI Adoption Is Too Expensive
The Misconception: They are prohibitively expensive tools for AI and available only to the largest enterprises.
The Reality: AI has never been this accessible before as the rise of cloud based solutions and scalable platforms makes it available to everyone. These affordable tools of AI cater to the needs of a specific SMEs as they cut down the cost and the value.
Myth 7: AI Lacks Transparency and Is Inherently Risky
The Misconception: AI has dark rooms, can’t explain or trust what they’re doing.
The Reality: However, challenges arise from the fact that these models are often opaque and cannot be justified or explained to users. To avoid the evil eye and abuse of criticism of AI, professionals must make sure that vendors provide transparency and use good governance frameworks.
Myth 8: AI Requires Advanced Technical Expertise
The Misconception: In fact, the most effective use of AI is only available to highly skilled data scientists.
The Reality: New modern AI platforms are getting easier and easier to use, with easy to use interfaces and automated workflows. These tools can be used by quality professionals without dependance on a deep technical bent, with emphasis on using the insights to drive improvement.
Emerging Trends: The Future of AI in Quality Management
However, we expect to see further integration of AI into quality management as trends like predictive analytics, autonomous quality control and AI driven risk assessments gain traction. In practical terms, roles of humans and AI will be defined by collaboration, and the humans will take up strategic oversight and innovation roles.
Conclusion: Embracing AI Without Fear
When debunked, these myths become allies of AI that quality management professionals can harness. Professional should not fear impact of displacement but instead should invest complementary skills such as data literacy, change management, and strategic decision making. The future of quality management is about AI innovation and empowerment of the human expertise to come together.
Read more on “Quality Management System for Artificial Intelligence (AI): Complete Guide for AI Developers“.
Actionable Takeaways for Quality Professionals
- Embrace Lifelong Learning: Learn skills in such areas as data analysis and AI ethics, continuous improvement methodology.
- Focus on Collaboration: Instead of thinking of AI as a rival to work against, think of it as a partner to help you do more and work smarter.
- Advocate for Transparency: insist the demand for explainable AI tools and more robust governance structures.
- Stay Agile: Ai technology evolves can adapt to new tools and methodology.
References:
- Effects of Artificial Intelligence on Decision Making in Project Management 2021, https://www.scirp.org/journal/paperinformation?paperid=107733
- Quality Management System for Artificial Intelligence (AI): Complete Guide for AI Developers 2024 https://hsquarehse.com/quality-management-system-for-artificial-intelligence-ai-complete-guide-for-ai-developers/
- AI Myths Debunked: True & Interesting Facts About AI 2024 https://365datascience.com/trending/ai-myths-debunked/
- Myths, mis- and preconceptions of artificial intelligence: A review of the literature 2023 https://www.sciencedirect.com/science/article/pii/S2666920X2300022X